With the presidential prediction game dreadfully same-y, surely there’s another constantly changing fix for political junkies. How about forecasting all 435 U.S. congressional races every day, based on brand new data every day?
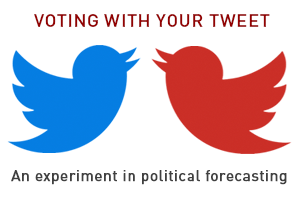
That’s what you get at “Voting With Your Tweet,” an experiment that mines mentions of congressional candidates in the Twitter-sphere to predict who will win each race and what the actual vote share will be. Unlike past efforts at using social media to predict political contests, which made their “predictions” after the voters had settled the matter, this research is happening now and you can check in at the California News Service site to see what’s up today, yesterday, or tomorrow.
Using social media as a forecasting tool is a hot area right now, and mixing Twitter with punditry is not brand new. Carnegie Mellon’s Brendan O’Connor compared tweeting to polling results in 2010, and not surprisingly found some correlation. Andranik Tumasjan and his colleagues at the Munich Technical University found that “the mere number of messages mentioning a party reflects the election result” in a German federal election. The “media utility” Tweetminster, meanwhile, says it accurately predicted the outcome of the last British Parliamentary elections using Twitter (an experiment they note is based on an earlier Japanese study looking at “online buzz and election results”).
Voting With Your Tweet is a kind of combination, using past results to predict future returns (but with mountains of caveats).
Analyzing about a quarter million tweets from 356 races in the 2010 mid-terms, UC Berkeley doctoral candidate Mark Huberty correctly “predicted” (after the fact) the winners 92 percent of the time, a much better showing than the six professional human pundits used for comparison. (The technical report on 2010 is available here, but be prepared to see that the 92 percent figure is one of a number of outcomes based on different statistical methods and statistical approaches. Huberty goes with “better than 85 percent accuracy” in the paper’s introduction.)
Taking that information, Huberty “trained two machine learning algorithms to determine what word features of those tweets best predicted whether the Democratic or Republican party candidate won each race.” So in its current incarnation …
We think the finished algorithm works like this:
First, it identifies from the language in a candidate’s tweets whether they are the incumbent or challenger. Since incumbents win about 85% of the time, this provides a good baseline.
It then adjusts the baseline prediction based on sentiment and action-related phrases. For instance, “voted hcr” (indicating that the incumbent voted for health care reform) was one of the most influential predictors alongside incumbency-related phrases. The algorithm weights those phrases positively or negatively, depending on how predictive they were of a candidate winning or losing.
And you can take this forecast to the bank? Only if you’re Lehman Brothers. “Voting with your Tweet is an experiment and should be treated as such,” Huberty and “data guru” Len DeGroot from the Knight Digital Media Center at Berkeley’s Graduate School of Journalism write in an honest and detailed set of FAQs. “We think this might work and think we might know why. But we could fail spectacularly.”
So why do it at all, or at least so publicly? The answers are refreshing:
Anyone who is interested can observe the experiment.
It keeps us honest: whether we succeed or fail, the predictions will be out there for all to see.
Observers can offer constructive feedback.
It gives us the opportunity post our own observations.
It offers the general public a window into the intersection of social data and political research.
So knowing this, I peeked at the putative winners for some races I’m following, starting with the competitive bout between Democratic incumbent Lois Capps and challenger Abel Maldonado in California’s 24th, Pacific Standard’s home turf. As of last night, VWYT gives it to Maldonado, with 53 percent of the vote.
Then there’s Berman-Sherman, the heated race between two Democratic incumbents battling for a single seat in LA? Ah nothing, not even Brad Sherman’s name, since the algorithm only codes for races with a Republican-Democratic contest.
OK, how about Kansas’s 2nd, the district where my mom is buried? (Kansas not being Chicago or Louisiana, Mom can’t vote there.) Incumbent Republican Lynn Jenkins is shellacking Tobias Schlingensiepen with a 65 percent prediction. That’s not surprising in this very blue state, but I wonder if the challenger’s name isn’t a bit Twitter-unfriendly.
As suggested, Huberty and co. see lots of ways this experiment can go pear-shaped, many of the potential problems hinging on using 2010 results to craft important topic choices this year. The mid-terms, after all, saw a powerful effort by Tea Party partisans, which could mean the terms over-represent Republican memes and challengers’ chops. Plus, there are new issues in the mix now, such as Libya and Solyndra.
Nonetheless, it’s fun to see political science in action and even better that for once it’s not about those two guys running for president.