If the machine uprising happened today, humanity would still have the upper hand; robots as we know them aren’t well equipped to recover from damage. Animals can adapt to injuries quickly, limping away mere seconds after an accident occurs. Modern robots, on the other hand, can take hours, even days, to learn new moves. But now, researchers have developed an animal-inspired “intelligent trial and error” algorithm that allows robots to recover from damage in two minutes or less.
“The most exciting thing is that it’s very fast,” says lead author on the study, Jean-Baptiste Mouret, an associate researcher in computer science at France’s Institute for Research in Computer Science and Automation. “Having [a machine] learn something in two minutes is very, very unusual in robotics.”
The team tested out the new algorithm in both a legged robot and a robotic arm. They report today in Nature that the algorithm quickly found a recovery option for all 20 damage scenarios the researchers threw at the two bots, including one where the walking robot lost two legs.
The robots achieved such quick recovery times because they didn’t waste time diagnosing the damage, according to Mouret. An injured animal doesn’t diagnose its sprained ankle; it finds a limp that allows it to keep moving. Similarly, the team’s robots didn’t pinpoint the damage; they just noticed a drop in speed or a change in course, and selected a new movement to resume their actions.
That selection process takes less time thanks in part to what the authors call a “simulated childhood.” Using a computer simulation of itself, the six-legged robot created a map of every possible movement pattern it could use to walk. (The robotic arm used a map of movements to drop a ball into a cup.) For the legged robot, that’s 10^47 behavior options—roughly equivalent to the number of molecules on Earth, the authors note. From all of those, the robot predicted which options would work best, testing them each out when the damage occurred. That way, when one gait failed the damaged robot, it had far fewer options to search through to eventually find a functional walk.
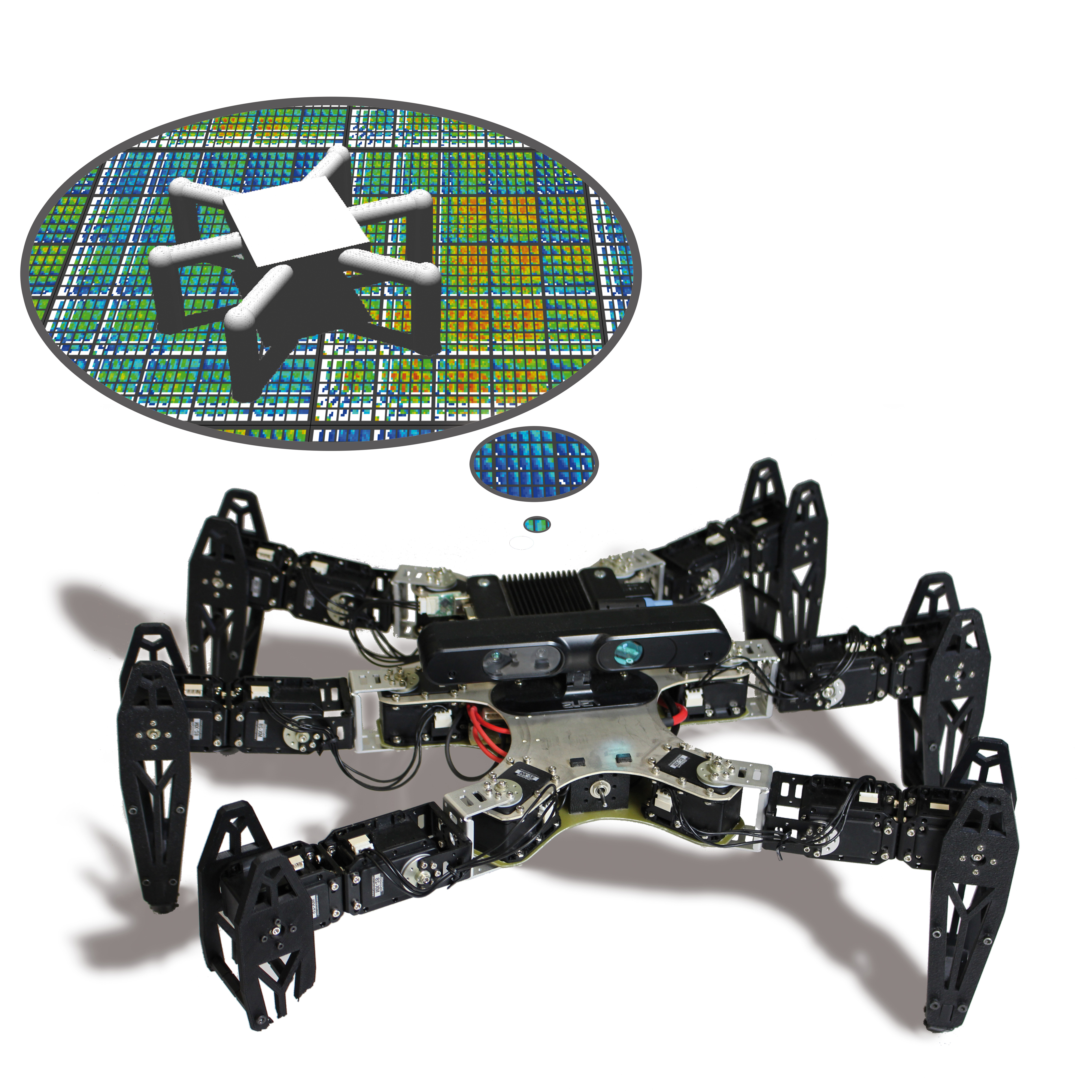
“In short, whereas previous algorithms searched for needles in fields of haystacks, we gather the needles ahead of time,” the authors write, “so we are searching through a pile of needles to find the right one.”
Thus far, the algorithm has only been used with robots in the lab, but the team believes it could make the real world’s (more advanced) robots better, and faster, problem solvers. Fast-learning robots could be useful for any situation in which machines need to function for extended periods of time with minimal supervision, such as natural disaster recon and rescue—environments where rescue workers can’t afford to babysit their machines. “We want a robot that can take care of itself,” says Mouret, “and won’t need to be rescued by the rescuers.”
Quick Studies is an award-winning series that sheds light on new research and discoveries that change the way we look at the world.