Sam Wang opens up about political forecasting, eating crickets on live television, and what we can all learn from Hillary Clinton’s shocking loss.
By Michael Schulson
(Photo: Justin Sullivan/Getty Images)
A month ago, Sam Wang called the election for Hillary Clinton.
Wang, a neuroscientist at Princeton University, moonlights as an election forecaster at the Princeton Election Consortium. He’s a poll aggregator, meaning that he runs the results of hundreds of polls through his model and then predicts election outcomes.
Wang predicted the 2012 elections better than pretty much anyone else, including Nate Silver. Since then, he has been among a handful of poll aggregators whose predictions make national news.
And this year, no one was more sure of a Clinton victory than Wang. Well before Election Day, he estimated her odds of winning at 99 percent. Wang was feted for this confidence; a short profile in Wired, published the day before the election, called him “the new election data king” and assured readers that, after Clinton’s victory, Wang’s methods would “be vindicated.”
When Wang called the race for her in mid-October, he promised to eat an insect if Trump got more than 240 electoral votes (he should end up with 306).
True to his word, Wang ate a honey-slathered cricket on CNN last weekend. After giving him a few days to digest, I called Wang up to ask what went wrong. We spoke about forecasting, hubris, and whether his overconfidence helped Trump win.
Why were the predictions so far off?
One [reason] is that this year that there was an unusually high number of undecided and third-party voters. And usually undecided voters will break approximately equally, but this year the evidence suggests that they broke in favor of Trump.
For much of the campaign season, it looked like there was a substantive bloc of Republican voters who were not supporting Trump. If you look at polling error, the polling error was worst in red states. And so you put all that together, it suggests that those party loyalists came home in the end.
Your model does account for shifts and polling error, though, right?
I made a mistake. I think the fundamental factor that made everyone wrong — and I should say, everyone wrong on both sides — was this polling error. I compounded that error by being excessively certain.
I underestimated the amount of uncertainty that was present in the home stretch. I thought that the polling error would be one point or less, and, in the end, the polling error was four points.
For all of the analysis that has gone into the Trump phenomenon over the past year and a half, it seems that, in the end, Republicans voted for a Republican. It was a fairly straightforward outcome.
As emotional as the race was, from a statistical standpoint there were many trends that ended up being pretty stable.
You called the election for Clinton on October 18th.
Yes.
How much was that an error of analysis, and how much was that an error of hubris?
That’s a good question. I will say that, based on observations of June through October, the race appeared to be fairly immovable, and the Clinton-Trump margin stayed within a pretty narrow range. I made a mistake in assuming that the home stretch would look like that.
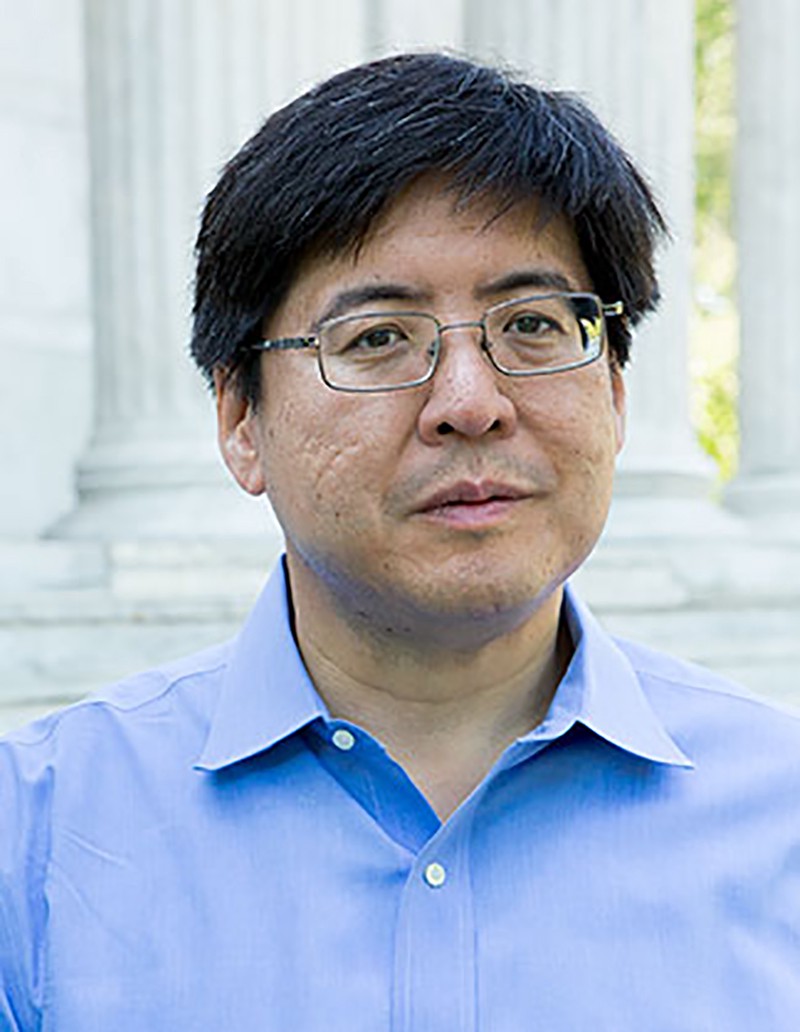
Sam Wang. (Photo: Princeton University)
Other poll aggregators have pointed out that, again, these undecided and third-party voters were significant sources of uncertainty. I was wrong about that, and I have to think about that and learn from it.
As you point out, pretty much everybody was wrong. But it seems like the key difference was how much faith individual forecasters were willing to put in their numbers. You ended up staking out an extreme position and saying, in effect, that you were positive the numbers told the correct story. Will this experience change how you think about the role of data in making predictions?
Yes and no. [My] hypothesis was based on [elections from] 2004 to 2012. State polls did a good job [in those elections]. Now the hypothesis is wrong, and I have to go back and face up to the new data in an honest way.
I think there are some obvious action items. One is to acknowledge greater uncertainty in the polling data that’s available.
Sure, the quality of the polls shapes the quality of the projections. But there’s a subjective element to that process of interpreting data and making statements about certainty.
One point is how to report situations that are uncertain. Probabilities are not a good way to convey uncertainty. The first reason being that it’s hard to estimate the true amount of uncertainty, and I discovered that. The second is that I think readers themselves are not necessarily equipped to interpret a probability.
So when FiveThirtyEight was giving a probability of a Clinton win as 80 percent, people will say, “A-ha, 80 percent is a done deal!” But actually, 80 percent is not a done deal at all; 80 percent is worse than the odds of surviving Russian roulette. And most of us would not voluntarily play Russian roulette.
A second point — you used the word “hubris.” I would not necessarily confine that to any one sector. I certainly take my responsibility, although I am a pretty minor figure. But people who report the news took for granted that Clinton was ahead. And that filled the tone of coverage.
Why predict elections at all?
Prediction is helpful in getting people interested in looking at the numbers. In the last few elections, [forecasts have helped] to put information in the hands of everyday readers about where to put their efforts.
I told readers that certain Senate races were close, and that if they wanted to make a difference, those were places they could [do so]. Those all turned out to be critical races for Senate control and also the presidency. So there’s a sense in which my advice was good.
Another use of polling data is to understand what different groups of the population think. A big story of the campaign was white voters who didn’t go to college being very supportive of Trump relative to previous years. Again, it’s a question of smarter use of polling data, and not focusing so much on who’s up and who’s down.
But even a nuanced take ends up getting boiled down into the simplest piece of information you can offer: who’s winning.
The challenge is how to come up with something boiled down that people remember that is not a numerical probability. I’m thinking pretty hard about simply not reporting numerical probabilities any more.
My poll aggregation was predicated on the idea that one could get rid of random noise and have a pretty clean measure coming out. And now what we discover is there’s a significant, uncorrected uncertainty. Even when one does an optimal job of getting rid of random noise, there’s still this correlated uncertainty that lingers. There’s an open question about what to do with that. I’m not ready to give up.
Do you think that you contributed to a narrative that helped get Trump elected?
The only thing that saves me from that conclusion was that I was a relatively minor figure. But it has occurred to me. I think a lot of people are engaging in self-examination now. I am among them.
What will that process of self-examination look like?
One question is “What’s the proper role of data in driving coverage of elections?” [Media analyst] Jeff Jarvis has criticized excessive focus on horse race. I actually have always agreed with him.
Despite being a data guy, my original hope was that poll aggregation would reduce the amount of media noise from individual polls and create space to write about issues. As it turns out, I think any review of media in the United States would agree that my hope was unfounded.
I don’t think it’s possible for me to run my little scripts and think of it as just operating within a vacuum, where all I’m doing is reducing data in the optimal fashion. I have to think about exactly how people read that data.
Sometimes knowing more can lead you to know less.
Well, yes, false certainty. The big lesson that I learned from this is to be more careful about engaging in false certainty. That’s a significant challenge. I have to face up to it. And I think that, to a lesser extent, anyone who engages in poll aggregation has to face the same questions.
You went on CNN and kept your promise about eating a bug. What has it been like for you to have journalists like me call you up and spend time talking about error? Do you wish that you could not talk to anyone for a few days?
It is obviously more pleasant to talk to reporters when the prediction is correct. I was not expecting to do what I call the inverse of a victory lap.
Crickets with honey was a classy choice.
Like I said [on CNN], it’s like John the Baptist in the wilderness. He ate locusts dipped in honey. If it’s good enough for him, it’s good enough for me.
This interview has been edited for length and clarity.