Science, it seems, has reached peak p-value. In research, the p-value represents the odds that your finding is a fluke, a coincidence, nothing more than a chance occurrence. In p-values, as in golf, lower is better. The pesky little decimal, usually found in a parenthetical following sentences describing a research paper’s findings, has been around for just one decade shy of a century, and in that time a significant p-value has become essentially a requirement for publication. And yet it says almost nothing about the size or strength of a result. But p-values, though flawed, aren’t the real issue in science; the problem lies in our expectations.
Regina Nuzzo explained the curious history of p-value in Nature last year:
The irony is that when UK statistician Ronald Fisher introduced the P value in the 1920s, he did not mean it to be a definitive test. He intended it simply as an informal way to judge whether evidence was significant in the old-fashioned sense: worthy of a second look. The idea was to run an experiment, then see if the results were consistent with what random chance might produce. Researchers would first set up a ‘null hypothesis’ that they wanted to disprove, such as there being no correlation or no difference between two groups. Next, they would play the devil’s advocate and, assuming that this null hypothesis was in fact true, calculate the chances of getting results at least as extreme as what was actually observed. This probability was the P value. The smaller it was, suggested Fisher, the greater the likelihood that the straw-man null hypothesis was false.
Jerry Adler first wrote about the problems with p-values for Pacific Standard in our May/June 2014 issue, when he observed an intellectual crisis unfolding in the social sciences. The scientific process—at its simplest, the formation of a hypothesis, the gathering of data that supports or refutes the hypothesis, and the publication of the results—was failing the field. The main problem: “Between the laboratory and the published study was a gap that must be bridged by the laborious process of data analysis,” Adler wrote. In that gap, scientists were performing what Adler called a “statistical sleight of hand,” manipulating their datasets until their results could be called significant. (In the psychological sciences, a p-value of 0.05 or less is usually considered significant.) This fiddling—whether intentional or not—is better known as p-hacking. And here is the real problem with p-hacking, according to Adler: “[I]f you can prove anything you want from your data, what, if anything, do you really know?”
Science is not a synonym for truth; it’s the process by which we search for it.
Adler’s question has since been underscored by a series of scientific scandals and misconduct that chip away at the public’s understanding of—and trust in—science. Perhaps most famously: a mishap earlier this year involving researcher Michael LaCour, whose heartening and widely reported study on the positive impacts that interactions with a gay person can have on one’s views of gay marriage was based on fabricated data.
But last week, FiveThirtyEight’s Christie Aschwanden reassured us that science is not, in fact, broken. “The state of our science is strong,” she wrote, “but it’s plagued by a universal problem: Science is hard—really fucking hard.”
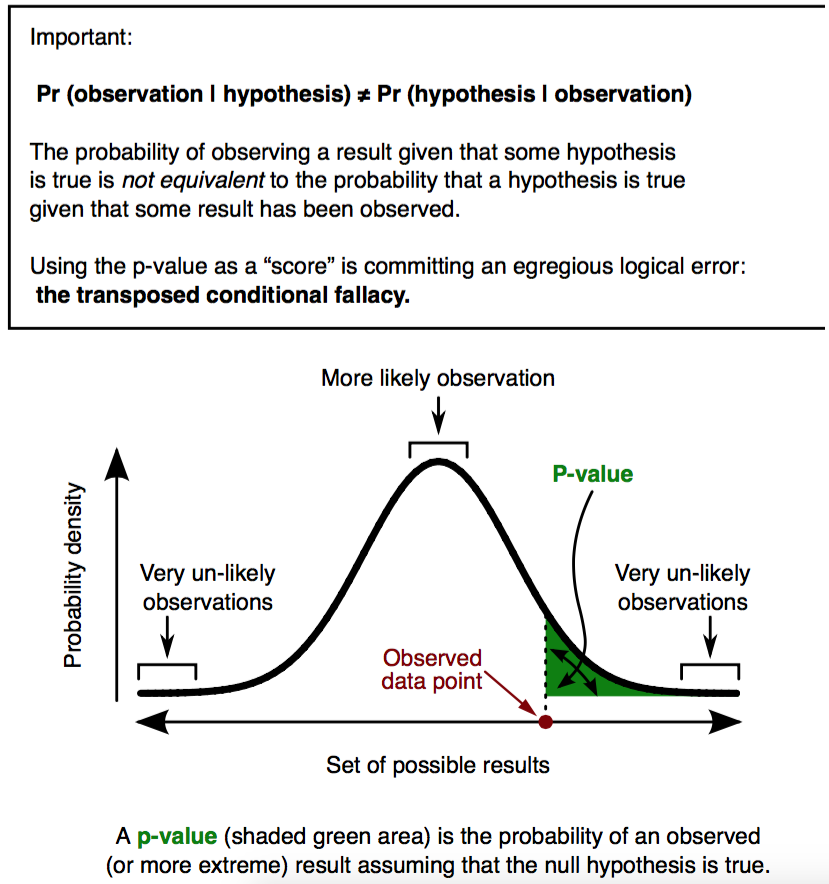
To showcase the complexities involved in statistical analyses, FiveThirtyEight created a brilliant infographic that allows readers to p-hack their way to a significant research result. The sample objective: Pick a political party and determine its economical influence. So, for example, are Republicans good or bad for the economy? The answer depends on which data you include in the analysis. The graphic enables you to include more politicians or exclude certain measures of economic performance, and watch as the data points shuffle into and out of discernible patterns on a graph. The data aren’t unreliable, but they are easily manipulated. As Aschwanden explained, nearly 60 percent of the 1,800 possible combinations of data in the graphic yielded publishable (read: a p-value less than or equal to 0.05)—and sometimes contradicting—results. So, are Republicans good or bad for the economy? The answer is: It’s complicated.
Both Adler and Aschwanden touch on other areas of the scientific process where misinformation and misdeeds arise, such as peer review, and present many of the same solutions: Research results should be replicated, peer review should be more transparent, and statistical methods should be more closely scrutinized. But all of these are just a partial solution, according to Aschwanden. The other side of the coin is something that Adler touched on only briefly. “We are living in an age that glorifies the single study,” Nina Strohminger, then a post-doc in social psychology at Duke University, told Adler. And according to Aschwanden, this means that our expectations of science are fundamentally flawed. Science is not a synonym for truth; it’s the process by which we search for it. Social scientists can’t save themselves because reforming the scientific process is only a partial solution.
“The important lesson here is that a single analysis is not sufficient to find a definitive answer,” Aschwanden wrote. “Every result is a temporary truth, one that’s subject to change when someone else comes along to build, test and analyze anew.”
Since We Last Spoke examines the latest policy and research updates to past Pacific Standard news coverage.